Charalambides-Christis aimed to leverage High Performance Data Analytics to understand the impact of various product offers on sales and costs. Although this is a frequent and well-studied business problem, in practice, the circumstances are unique for every company and situation, requiring a tailored approach to accurately assess the impact.
The primary goal was to identify the average treatment effect (ATE) of an offer on a product. ATE is defined as the difference between actual sales and what sales would have been without the offer. Since ATE involves a hypothetical scenario—sales in a world without the offer—one way to approach this is by creating a machine learning model that learns from instances where no offer was applied to the product.
We recommended developing a machine learning model specifically designed to predict time-series data for supermarket sales. The model would leverage input features such as seasonality factors derived from timestamp data, weather conditions, and the sales of milk—a product without promotional offers that can serve as a bellwether. Once trained on data from non-promotional days, the model can be effectively applied to forecast sales on days with offers. By comparing these predictions with actual sales, it becomes possible to estimate the Average Treatment Effect (ATE). The following graph illustrates our approach:
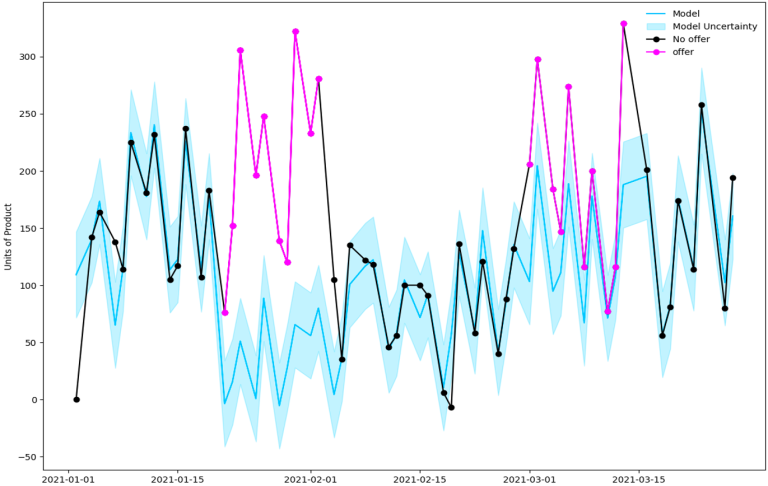
This is what George Mouzouras, Information Technology Manager of Charalambides-Christis, commented about our work:
“We Collaborated with CaSToRC within the framework of the EuroCC2 project, to calculate the Average Treatment Effect of our product offers using advanced computational methods and High Performance Data Analytics.
This professionally executed and well-documented collaboration, not only provided robust technical consultation, but also unveiled new opportunities for leveraging state-of-the-art technology in our analyses.”